Business innovation through AI-Tech
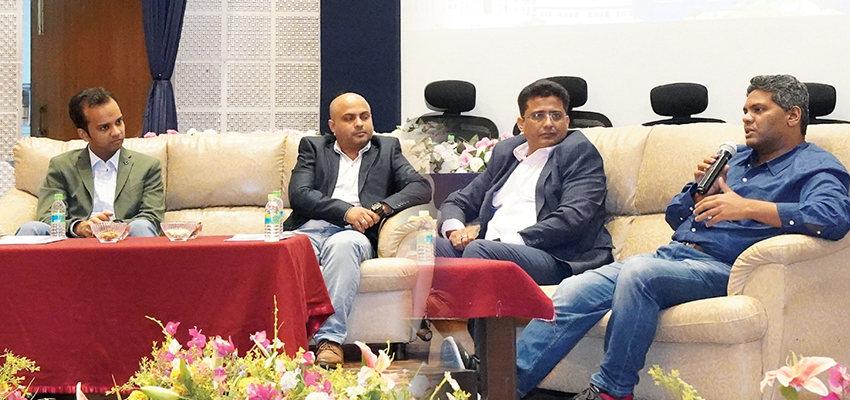
Artificial intelligence and machine learning, combined with human intelligence, have significantly transformed corporate work. With the induction of AI-driven solutions, companies are now able to reach new heights of growth and efficiency. In a recent panel discussion on "Innovating Business Through Technology and AI-Driven Solutions" held at Sri Balaji University, Pune (SBUP), the distinguished industry experts discussed the deep impact of AI-driven solutions on the corporate world, which has completely changed the manual working methods. The panellists were Shashi Shekhar Tripathi, co-founder, eShipz; Gaurav Kejriwal, vice president, Exl Analytics; Udit Paliwal, food and agri practice, KPMG India; Chetan Dixit, director-product and data platforms, VISA, moderated by Sana Ansari, senior assistant professor-business analytics, SBUP. Corporate Citizen brings you excerpts from the discussion
Sana Ansari: What is the role of AI and technology in your organisations?
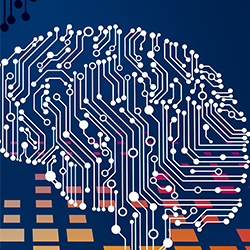
Gaurav Kejriwal: With the advent of generative AI or AI-driven solutions over the last few years, EXL services have been developing a lot more customer-centric solution drivers that cater to every problem. You can directly put a query on a system, and it will generate a presentation and visuals in a minute. For the last couple of years, we have built prototypes, and we are seeing a lot of fractions in the industry.
AI is changing the world in the operations industry. When agents talk to their customers, the amount of time taken by agents to solve a query is large, or they are not able to personalise their communication based on the requirements of your questions. So that is where generative AI or AI-driven solutions come into play. These solutions assist the agent with relevant topics and relevant personalised responses to the questions in real time. They can summarise the calls in a minute and analyse what went right and what went wrong there. AI solutions can also identify the sentiment of overall communication that is happening.
Shashi Tripathi: We provide an intelligent platform for big corporations, who dispatch a lot of materials—dealers to vendors, dealers to distributors and consumers. Since the dispatches are lakhs in months, we provide an intelligent platform to find the right service partner for them. It could be based on pricing, services and many other factors. Companies need a tool to help them identify the right information and the right service partner.
The companies are also facing a lot of challenges in the e-commerce industry. Several people order a lot of e-commerce products, but they never accept those products, and they end up cancelling those orders. Here, the AI can identify a person who cancels the order every time. After identifying such customers, these AI tools help the company reduce the cost of logistics. We help companies identify these loopholes and make better decisions to increase supply chain efficiency.
We use artificial intelligence technology to dig deeper into the data available and do modelling so that we can arrive at a scenario of planning to understand what kind of scenario will unfold if we apply one kind of solution to another
- Udit Paliwal
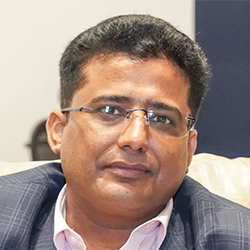
Chetan Dixit: I will take the example of OLA to illustrate the use of data for our day-to-day tactical operations from a strategic point of view. Today, in the e-commerce world, a lot of decisions have to be made, and now you don’t have the time to take the decision. Here, these machine learning(ML) algorithms are coming into play to make these pricing decisions. For example, if you are in the Hinjawadi area and book a cab to go to the airport, how does it dynamically decide the price? So, it is simple economics; it’s about what is in demand in that area and what is available in that area. But for me to garner that amount of data that has been scaled and then processed, the pricing decision is where a lot of these ML algorithms are kicking in from behind.
Another example I want to add is about the Visa company, where we process payments for millions of users globally, primarily in terms of fraud detection. In the banking and financial world, it is really big because an undetected fraud costs the company hundreds to thousands of dollars. Here, ML algorithms and data in general, can detect fraud and prevent fraud and fraudulent patterns.
Udit Paliwal: We implement human intelligence with artificial intelligence. Because in the consulting world, you can have several problems and several solutions. But with the kind of complex ecosystem in which the businesses are operating, there are loads and tonnes of data being generated by the ecosystem. All this data is being thrown to the management, and they cannot process it using their human intelligence. Hence, this data needs to be supplemented by data analytics or some artificial intelligence so that the right problem statements can be identified.
As a consulting firm, we deal with clients whose projects are worth a few hundred lakhs to the government and large corporate clients who have a few thousand crores of business or projects at a time. So the first thing we do is use the available data to identify the unique problem statement. This is where we use artificial intelligence technology to dig deeper into the data available and do modelling so that we can arrive at a scenario of planning to understand what kind of scenario will unfold if we apply one kind of solution to another.
The second thing is to customise the solution. For example, there are clients ‘A’ and 'B'; their business sizes and problems are the same, but the solution might be very different for each of them. So this is where technology is driven. The third thing is how we create a customer solution and customer engagement with our clients; this is where we use the technology.
Q: How has the availability of data impacted the fields of business and innovation? How does an organisation manage fragmented data?
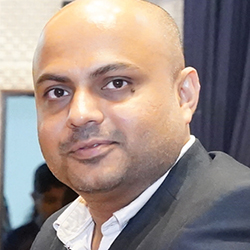
Gaurav Kejriwal: Every client is at a different maturity level when it comes to data and analytics; some of them still maintain data and excel sheets, while others have just begun building that data infrastructure on premises with their physical hardware. On the other hand, some of the clients are already in the cloud, where AI applications are much easier. Therefore, for a consulting firm, it’s very important to understand where a company is in its maturity level and go with the right solution accordingly.
Udit Paliwal: I want to illustrate with an example from the agriculture sector. I closely work with the secretary and the joint secretary in the Ministry of Agriculture and Farmers’ Welfare, Government of India. While working on a project, a major problem that we face is the crop production data—the land holdings are so fragmented and the land records are also outdated. The second challenge we face is the kind of data that the government gets. So, what we have been suggesting to the government is to adopt AI and ML based data collection so that the reliability of the data can be increased.
The use of technology is extremely important in terms of getting the right count of the data. Because, whenever there is a human intervention, we assume that there will be errors. As humans are made to make errors, we use AI with human intelligence, because both of them complement each other. Both are required to do a lot of work with the data collected, and through the technology we refine it with the use of humans.
Chetan Dixit: An organisation's maturity is determined by how efficiently they manage their data. If there is siloed departmental-level excel sheet-type data management, then they have a lot of maturity. You’ll have a data asset for every aspect of the business, for every entity of the business, including your customers and your vendors. These are well-governed data sets associated with good data quality. Organisations understand this much later in their maturity curve. Data is the foundation, and data assets are the very core, and that’s how most companies will propagate in terms of the maturity curve.
"There are a lot of tools available, and change management is required, but at the end of the day, it is all about how and what we are entering into this system"
-Shashi Shekhar Tripathi
Shashi Tripathi: For any supply chain team or any pickup team, at each pickup point, a lot of things happen manually. In terms of digitisation, a lot of big companies either lack or have some restrictions at their end or haven’t implemented any kind of digitisation. So the person will operate with a manual work process for thousands of clients, including writing things on paper. Hence, all this manual process needs to have manual errors, which is again a loss to the company. Now, using a few applications, the companies are not only able to work digitally but also complete the work in a fraction of a second. Now the large companies have enough teams to go through all those papers, whether they are signed, stamped or not. But, with certain tools having OCR technology, by identifying them one by one digitally, it can take some actions on very short notice as opposed to going through multiple stages.
Q: What metrics do organisations trade to measure the success of AI-driven solutions?
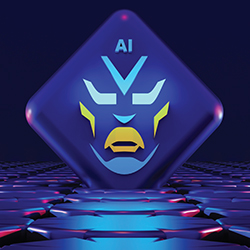
Shashi Tripathi: Nowadays, companies do ask for a lot of digitisation, but those are more like a wish list. The company may end up investing a lot in those implementations and may not use them because it’s more of a change in management. Some of the large giants like Maruti Suzuki, PhonePe and many other companies have had their team members work in the organisation for the last 20 years. If you want them to change their approach, then it is a very difficult task.
Now that the data is right, everyone is doing all the necessary things. We try to have a KPI (key performance indicator) set for ourselves and share it with our clients as well. We say that if you want this piece of digitisation, this is how it will work, and this is how we will work in collaboration. So, I do a part of it and my system will do the validation, but you will have to give the repository validation, which says if it’s matching with your systems or not.
For example, someone has dispatched 1000 packages or 1000 shipments over two months, and 60 percent of the PoD (proof of delivery) is available and 40 percent is missing. So why is it missing? We work together with them to see if we can improve the systems because they cannot work if someone is not acting. So we have to nurture the data; we have to clean the data to make it better.
Gaurav Kejriwal: When we talk about AI investment and the impact on top of it, we have to understand that investing in AI is not just a digital transformation that a company is making; it’s a cultural transformation as well. You have to train your employees and help them understand why this digital transformation is happening. Because if they are not supportive or aligned with their strategy, you will not reap results at the end of the day. Any AI investment will have an objective in mind and that investment cannot work all alone. The rest of the business function should work together with it, and at the end try and see whether you can achieve your objective, even in parts or not. So, it’s not just a particular investment that you are making; you are transforming your entire business.
Chetan Dixit: In the organisation, we had very clearly defined business objectives and implemented AI. Artificial Intelligence(AI) is not just a fancy term; it is of no use if it cannot solve any business problem. I should be able to increase my revenue, reduce my costs, get more rides and get more people to spend because I’m part of a credit card organisation. So there has to be some very clearly laid-out business objectives that you start with. You have to very clearly have mathematical formulas around it so that at the end of six months of tuning, maturing and training the model, you know how close you are getting to the business objective.
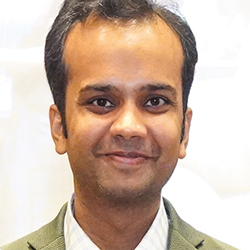
There is also a lot of investment behind the scenes that goes on in terms of data collection, data massaging, correcting the data, etc. It takes people and tools to get the data and the model, and it’s a continuous process, so you are investing a lot of people, hardware, and software licenses. But is it generating the desired return on investment? You will only be able to answer these if you know what you set out for to begin with.
Udit Paliwal: In our case, there are two scenarios - either you are risking that you are not implementing the right strategy (from a client’s perspective) or you’re risking implementing the wrong strategy. Here, data analytics and data mining help the management make the right decisions and develop scenario planning and mapping. We help our clients understand the cost- benefit analysis of implementing a particular technology vis-à-vis the return they are going to get.
The technology can help in predicting a scenario, creating a model where the management can foresee the situation and make an appropriate decision. It helps the company with closer monitoring of all the expenses because they can get all the information on their dashboard at their fingertips. They don’t have to call a meeting for that—so we can develop dashboards wherein they can see which functions have a maximum cost versus the returns that are coming. So, this is how we are helping our clients identify and understand the problems well in advance.
"AI, or AI-driven solutions solutions assist the agent with relevant topics and relevant personalised responses to the questions in real time."
- Gaurav Kejriwal
Q: How do you access the scalability of the AI-driven models?
Chetan Dixit: In my organisation, we are running the world’s largest financial data platform. Daily, we have about 500 to 600 million transactions—at least 500 million times a card is swiped somewhere in the world, which leads to the generation of huge amounts of data and scale problems. Here, the scale of the problem is not just in terms of storage, data reliability and quality, but also the scale of the model. Scales present a lot of challenges and a lot of opportunities, depending on how we manage them at a global level.
For example, if you swipe any credit card or debit card, a lot of times you are asked to authenticate whether it is you who is performing the transaction. This whole authentication is an AI or machine learning model, working in the background. However, the ability to detect this at scale for every transaction is the main challenge. So here we play around with the risk score for a transaction because something different happens. I will increase the risk score for that particular transaction to 0.7—a typical score between 0 and 1. Still, there is a 70 percent chance that this transaction could be fraudulent. So, what I will do is bring another layer of authentication where my ML will send an OTP to the mobile phone of the user and say, "Please confirm it's you"—this is called two- factor authentication. If the OTP authentication fails, then I’ll decline the transaction. This is where scale models come into play and solve a real-life problem for you.
Gaurav Kejriwal: Scalability is a problem for several users; it even causes a problem, especially when you scale from an English speaking country to a Japanese country. So in English, we have 26 characters, but in Japanese, we have 15,000 characters, and if you combine 5 to 6 characters based on how you are speaking, the meaning can change with just that combination. In the same combination, the meaning could be different. When our clients were already working with English speaking countries as well as Japan, there was one person who had 26 different cards with the same bank, and he had defaulted on 20 of them, and his application for the 27th was also approved, because he was just changing the way he was writing his name. At one point in time, he is writing in a different script, and the other is in a different script. He was mixing and matching the script and just going ahead with it. Here, scalability is important because when you move to a different geographical location, you understand the needs of that market and the needs of that language. You have actually either built that algorithm again or retrained your existing model.
Retraining is not only important when you are expanding to a larger population but also because scalability means reaching out to the larger population you are working with. Your business has also been running for years using the same ML methodologies; the same algorithms cannot work for years as well. You have to keep it updated, and that’s where scalability has its challenges
Shashi Tripathi: In our industry, we work with a lot of semi-skilled people who are using the applications. The problem statement that we have seen with these larger corporations is that they were working from a big warehouse where the internet was not proper, hence the data itself gets nullified.
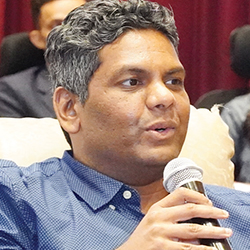
Hence, we asked the companies to provide some kind of solution that would help them from an internet point of view. The company started looking into digitisation. Earlier, the courier staff and team were managing everything manually. For example, if some item is to go to Fort area in Mumbai, pin code 400001, the person goes to write with a pen. So at the end of the day, if he is dispatching thousands of boxes by the time he finishes the last set of boxes, he will write zero, which will look like six. Hence, the data entry in the system, which is a manual system itself, is wrong. So when someone is looking into that, he will enter his system as six. So the dispatch will be initiated in Thane or Mulund (in Mumbai) instead of going to Fort.
There are a lot of tools available—change management is required, but at the end of the day, it is all about how and what we are entering into this system. Only then things will read right and we will be able to churn those data, do validations and many other things.
Udit Paliwal: We are 1.4 billion plus people, and probably those who are not connecting with technology may not be more than 30 percent. And even if you go to the government, there are a lot of processes that are yet to be optimised and automated. I’ll illustrate with an example of an HDFC bank and contrast it to how the government operates. Most of the credit proposals that are limited to the HDFC bank are scanned at the first level, and the decisions are taken by their AI or ML based tools. So it’s not humans who are doing credit appraisals for HDFC Bank; vis-à-vis, if you come to the government, here if you are applying for any document like a death certificate or passport, humans are sitting there scrutinising your documents and then giving a go-or-no-go decision. This is the area where a lot of scopes are available in terms of the process of automation , the process of optimisation and risk mitigation. Considering this, the consulting firm is making heavy investments in AI and ML capabilities. They are also doing a lot of capacity augmentation in terms of technology experts. There is a lot of scalability; all the major consulting firms are making a lot of investments, including Grant Thornton.
"In the banking and financial world,an un- detected fraud costs the company hundreds to thousands of dollars. Here, ML algorithms and data can detect fraud and prevent fraud and fraudulent patterns."
- Chetan Dixit
CC: What is your advice for the young generations? What are the skillsets they require to fit in the organisation?
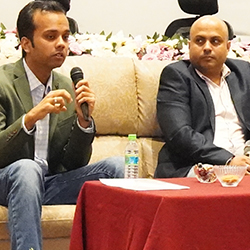
Chetan Dixit: Multiple job streams come together to make data work for the business. Someone with an engineering background will usually become a data engineer. So the skill set of a data engineer is to build pipelines. Data engineers are the people who build data assets or data products that will stand the test of time. Then comes your business intelligence generally called data analysis. Being a data analyst requires the ability to efficiently write a query on a data system. A database could be a sequel document, and query-writing skills are crucial at that level. Then you also have business intelligence folks who come in and can create dashboards and reports.
Several organisations are also creating a new profile called Citizen Data Scientist, which is a real rule. It involves the final frontier of dabbling with models, creating models, training models and making business decisions.
No matter at what stage you are operating, you need to be a great storyteller. You need to have the ability to define a very clear narrative because you will be presenting this data to somebody who is hired in the organisation. You should develop those articulation skills in addition to your data skills. This is missing for most engineers, but those who come from non-engineering backgrounds are a little more creative, and they can at least churn out a story. But, no matter what job you take up, whether you are a data product manager, a data analyst, a business analyst, or an ML scientist, develop the ability to narrate a story through data that will appeal to your stakeholders.
Gaurav Kejriwal: The skills I would suggest are persistence and problem-solving. Any data or any format will not give you answers until you are very persistent and are trying to identify insights from it. Sometimes, on the face of it, you are not able to identify any trend or pattern, but if you are persistent, you will get something out of it.
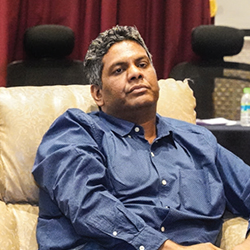
When it comes to problem-solving skills, you will face a lot of challenges in whichever stream you are in. You will face a lot of challenges and nothing will be served on a platter. You should be able to find the root cause of the problem and solve it by trying different methodologies.
Lastly, the learning attitude—this analytics space is ever-changing—you may work with a lot of different businesses, lots of different domains, and have different problem statements. So, try to develop that learning attitude and keep yourself updated with recent trends and advancements so that you are relevant in the overall ecosystem.
Shashi Tripathi: In my point of view, the analysis starts when someone is open to doing the analysis. Many times, companies are individuals who have seen some sets of data, and they have made some kind of module into their systems that will read the data. However, the company for whom they are working may need this data in a different format to be analysed. So analysis is always best when you are open to starting from scratch.
For example, if you are working in the automotive industry, there is always a different need for each customer. So unless you are open to changing according to the modules of the company, things may not work. There is no need to be super advanced or become someone who can do Python coding—data engineers can do it. You can be an analyst, and analysts can start from zero and then they can build up the stories, learn from the company, take some inspiration from outside that is readily available in the market, and then modulate it accordingly.
"We use AI with human intelligence, because both of them complement each other. Both are required to do a lot of work with the data collected, and through the technology we refine it with the use of humans"
- Udit Paliwal
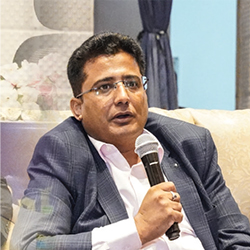
Udit Paliwal: From a consulting point of view, there are quite a few traits that are very critical—personal and professional grooming. Whenever you are going and meeting a client, you should always be one notch up the client. If you are three times up with the client in terms of personal grooming or dressing, the client will get offended and will not accept you. If you are one notch below him or on par with him, he will take you for granted. So, you need to have a personal grooming plan in place wherein you need to look a notch better than a client.
Further, in terms of professional grooming, you need to have more information, solutions, and understanding than your client can have. The client is a master in his field and when he calls you for a solution, he knows the problem and probably knows the solution as well. So professional skills-wise, you should be at least
a notch above him. Besides this, two things you should always keep on doing are exploratory travelling and exploratory reading. Both of these will help you add value to your personal and professional outlook.