AI in Indian BFSI
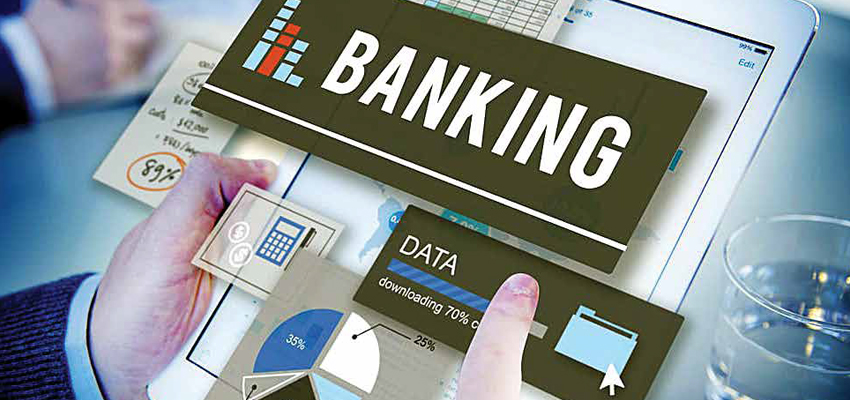
Artificial Intelligence, IoT and Blockchain are emerging technologies that are impacting various aspects of human life. Among these, AI is arguably the most ubiquitous and disruptive in nature. The use of AI by organisations and governments and its deployment in improving customer experience, operational efficiency, fraud detection and cybersecurity have all been on the rise across the world. To understand the true impact of AI on the Indian banking sector, CII conducted a panel discussion titled ‘The State of AI in Banking and Financial Services’, moderated by Dr Koustuv Dasgupta, Head, Goldman Sachs R&D, India featuring an elite panel comprising of Shalini Warrier, Executive Director, COO and Business Head – Retail, Federal Bank; Mrityunjay Mahapatra, Member of the RBI innovation Centre, and former MD and CEO, Syndicate Bank; Nitin Chugh, CEO and MD, Ujjivan Small Finance Bank and John Mathew, Founder and CEO, Riafy. The panel sheds light on the pace of AI adoption in Indian BFSI, the challenges encountered on the way and the opportunities that lie ahead
Although the adoption of AI varies significantly across geographies, there are an increasing number of pockets in industries that are adopting AI to provide better service to customers and bring efficiencies in their processes. One such industry is the Banking and Financial sector. AI is demonstrating a huge impact on processes, products and user experience in the BFSI. Developing AI infrastructure is becoming a key priority for the Indian government.
"My sense is that the change is much faster than gradual. There is a dilemma that banks are going through. Their margins are under pressure and therefore, there is a need to keep costs under control"
- Shalini Warrier
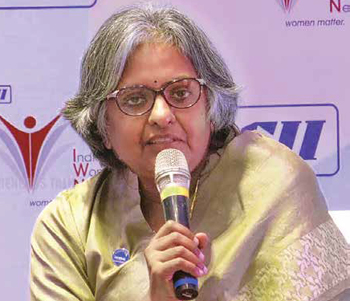
Dr Koustuv Dasgupta: Do you see a gradual shift in the Indian banking sector in the last few years with respect to AI?
Shalini Warrier: My sense is that the change is much faster than gradual. There is a dilemma that banks are going through. Their margins are under pressure and therefore, there is a need to keep costs under control. Then there is the customer side of it. Today, customers are demanding a level of service from banks on par with what they are used to experiencing with Uber, Swiggy and Amazon, but with the trust and security of banking. People want a seamless user experience. AI appears to be very well placed to take us forward on that journey. Virtually, every bank has invested in chatboxes so that customers don’t have to wait in call centre queues. There is also the question of financial inclusion. How to get more people into the banking sector, not only through the Jan Dhan accounts, but far beyond that. Then there is the investment advisory area that is adopting AI. Now you don’t need to depend on an investment relationship manager, you can deal with a bot who can give you all the responses. Also, there is the aspect of personalised experiences for customers. What I want is different from what you want. Broadly speaking, I feel AI is here to stay, and it is here to deliver benefits.
You led the digital transformation at HDFC Bank. In your experience, what are some of the challenges that banks face?
Nitin Chugh: I think that there needs to be a roadmap for AI. AI is not plug and play. AI is certainly not something that can be invented by banks. Banks need to work with people who understand that domain much better than them. Logically, we are starting with simplistic work cases, such as-How can AI help us perform certain functions better? How can AI assist in our workflows? The second phase, which we are coming towards as an industry is how does AI augment our capabilities? Can you assign certain tasks to AI applications and leave them alone? The third phase is the autonomous phase, where you leave the whole application alone and it functions by itself. It generates insights and also creates a call to action. It takes decisions, it learns and it gives you the outcome the way you want and when you want.
I think we are very far from this phase at the moment, especially in BFSI, and I don’t think we should be rushing into it. My learning has been that we need to take small steps, even though the technology is advancing very quickly. A lot of this work that we are trying to do in the industry is based on data. But if you can’t make use of the data because it isn’t contextual or relevant or unstructured and you cannot convert this data into a call to action, then it's best to look at what your core data tells you. Collectively, we still have to do a lot of work in using the data that we already have and making sense of it, because too often we come across instances where the information is with the bank but the bank hasn’t taken action. We first need to look at these capabilities which are lying at an underutilised level and work on those.
"Too much of hype puts real talent away. Keeping it simple, starting small, scaling it up, building private collaborations and putting the eco-system right is the way forward"
- Mrityunjay Mahapatra
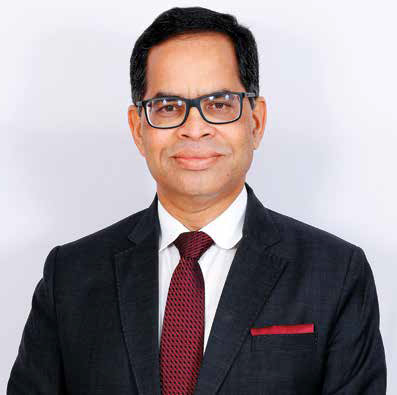
There is no dearth of investments in AI transformation. Where do you see the Indian banking sector in this paradigm shift, and how is the RBI innovation centre playing a role in using technology breakthroughs to bring innovations to the Indian banking system?
Mrityunjay Mahapatra: I must first clarify that we are still setting up the RBI innovation hub, and the views that I give here are my personal views as we have not brought out a policy in that regard. I tend to agree with Nitin that AI is more hype than reality in the Indian banking sector today. It has become fashionable to speak about the application of AI, whereas in effect, what they are doing is putting together a few algorithms and augmenting them into a workflow and getting some results in the form of a chatbot or a voice bot and getting a few simple problems solved. In my view, today the Indian banking AI problem is a plumbing problem. The plumbing has to be set right.
The first problem is the problem of data. What we are seeing is that data is multi-residence and multi-protocol. Data is stored in different logical formats in different ways. We do not know how we will use data in a connected ecosystem where, giving a loan will probably involve not just fetching banking records, but also the land records, medical records and insurance history. How do we go towards that? The second plumbing issue that we are facing is that like any developing country, the regulatory and policy framework is a work in process. It is good to think big, but I feel private collaboration in the AI field is the way to start before we go into a countrywide AI protocol. The problem with the banking sector is that banking is getting increasingly connected, and as we travel from products to channels to platforms to experiences, I think what we need to do is that in addition to these transformations, we will have to look at the appropriate technology which will be secure. Too much of hype puts real talent away. Keeping it simple, starting small, scaling it up, building private collaborations and putting the eco-system right is the way forward.
"I think instead of talking about other aspects, we should get back to the fundamental use case of what a customer wants. What feature will make the life of the user easier? I think if you flip the thought process, it will be a lot easier to look at AI as a space that will work"
- John Mathew
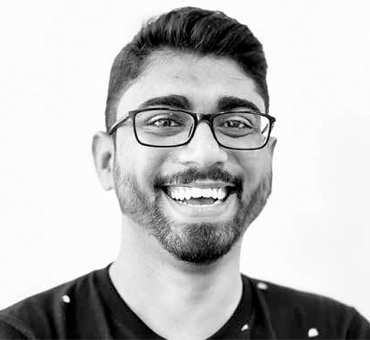
When you look at all of the hype as well as the reality on the ground, what is your take on the direction that we are moving? How should we be treading on this journey?
John Mathew: What we did in the last 5-6 years of our startup journey is to use AI to help people eat good food. Using the customer experience to predict what people will like to eat, we pitched this idea to Shalini ma’am. She asked us if it would work. Everybody is sceptical because AI is this word that is thrown around loosely. That is a major problem. When we talk about low hanging fruit such as answering a customer’s query, there are two parts to it. The first is understanding what the user actually meant and the second one is the ability to find an answer to that. What is currently missing in the banking eco-system is the ability to understand the question and search for an answer from the banks' archives.
I think instead of talking about other aspects, we should get back to the fundamental use case of what a customer wants. What feature will make the life of the user easier? I think if you flip the thought process like that, it will be a lot easier to look at AI as a space that will work. Currently, everyone is trying to make AI very fancy and make it do a multitude of things. All of this will require you to use large scale processing of data. There are security issues with this process. Our thought process is simple. We do on-device machine learning. Why don’t we forget about the servers where user data exists? That data belongs to the user. Why can’t we process the data on his phone and give him insights. The perspective on AI in terms of banking applications should be shifted from the traditional aspect of having all the data on servers and processing it in order to create something that the user wants and needs.
"My learning has been that we need to take small steps, even though the technology is advancing very quickly"
- Nitin Chugh
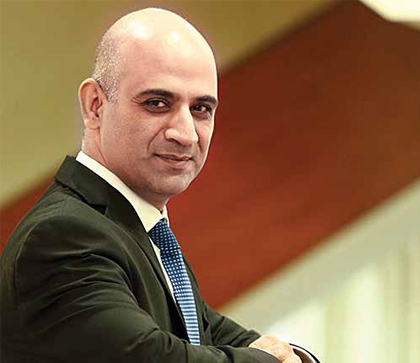
Are we ready with the skills in the BFSI sector in India to understand and interpret results?
Nitin Chugh: I was at my engineering college a couple of years ago, addressing the first-year students, and I was telling them that the generation prior to us worked on the hardware systems, we are the generation which worked on the software section, and you are the generation which will work on data and AI. I think the older generation does not have the skills. We can try and reskill and try to build new skills, we can try to trade a hardware engineer for software, but we won’t succeed to the scale that we would expect. I think we need to start bringing in that curriculum itself where we start training the next generation of engineers to be able to work on those application tools, otherwise, it is too much of an overload for people to unlearn and then learn.
If you already struggle to make sense of an excel sheet, what hope do you have of making sense of data without any basic knowledge of coding? Now we know of children getting enrolled for coding at the age of six or seven itself. Coming back to the present, I do think that we have a shortage of skills. We don’t just have a shortage of data science capabilities, we also have a shortage of interpretation skills. But the good thing about experience is that the decision making is very nuanced. People who may not have the tech skills are able to make those decisions because as long as they have the information in front of them, they can make a decision whether to move in Direction A or Direction B. I think that is something which is very valuable right now. These experiential skills also need to be passed on to the next generation. At the moment, we are going through a phase where every time there is a generational change, you have a blend of the old and the new, and then you have a phase of the overlap. We need to work on a very clear skilling strategy across the ecosystem.
What efforts do you think we need to take to ensure that there is a steady availability of skills and talent and the next generation has the necessary tools and interpretation capabilities to take us forward?
Mrityunjay Mahapatra: This is a very relevant question. I will take it from a very structural perspective. Anything to sustain all the four pillars of a concept must move in tandem. I call these pillars People, Process, Technology and Governance. As the adage goes, the strength of the chain is the strength of its weakest link. In my experience from my long years in the US and UK, one of the critical success factors is a seamless move from academia to industry to government and vice versa.
When I was at SBI, I started a collaboration with IIM Calcutta. The results were fabulous, because students who are academically oriented want to come and work with the industry to test their own learning, contribute to the industry and go back. I say we must facilitate this. There are three or four use cases where I believe the application of AI can happen quite quickly. One of them is the early warning signal for loans and compliance errors. The second is reconnaissance, and the third is anti-money laundering. AI can be very useful in dealing with false positives. Processes of AI deployment must be strengthened. Technology should be collaborative between people who do technology as a profession. Domain expertise must come, because a lot of startups fail at the edge of scalability. We need to find a way to make AI scalable. These are the real problems that we are seeing.