Decision Making Under Uncertainity
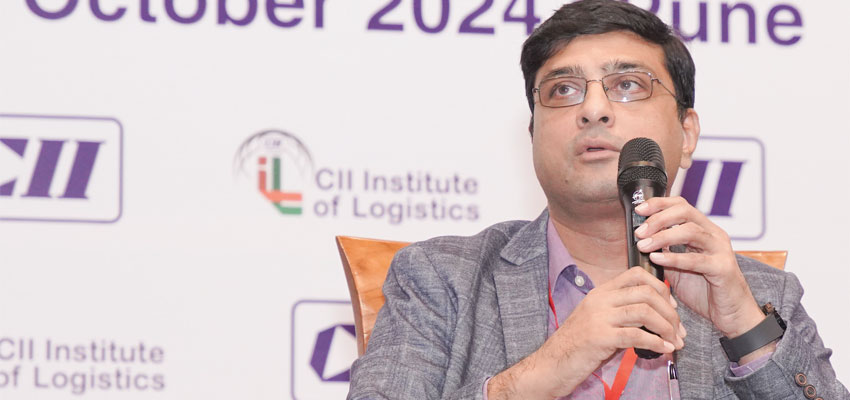
Supply chain management is important as it helps businesses to reduce costs, choose efficient and cost-effective suppliers, and timely deliver products to customers. Supply chains can be affected by many known and unknown factors including material shortages, increasing costs, unpredictable demand and natural disasters. Decision makers while taking decisions under uncertainty must plan properly so that their decision reaps benefit for their business and not adversely affect it. Addressing this topic a fireside chat on the topic “Decision Making Under Uncertainty” was recently held at a conference organised by Sri Balaji University, Pune (SBUP) in collaboration with Confederation of Indian Industry (CII), at the University campus. Corporate Citizen brings excerpts of this chat
Shammi Dua: It is difficult for manufacturers to predict customers and shoppers buying behaviour, as they have much more options today. Who is going to buy what, when and from which channel, is the question. Then there are external uncertainties - weather, dollar price, crude or geo-political solutions and suppliers. We have learnt a lot in the last 3-4 years since Covid pandemic. So, there is lot more uncertainty in the current times and it's only increasing. What is this decision making in uncertainty?
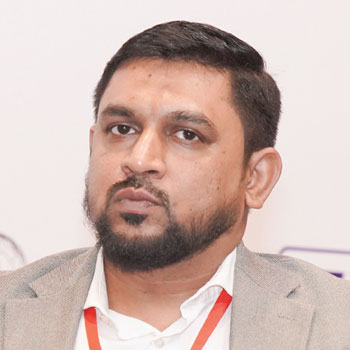
J Venkateswaran: As this term suggests, we are trying to make some decisions, and we are not fully sure about its outcomes or some of the parameters within the system that are occurring. We are not sure, but we are still forced to make decisions. This is better understood in context when we know everything. For example, if I drop this microphone, then it is certain that it will fall down under the influence of gravity. So, there is no uncertainty to think or worry about. But then imagine, what if everything is known. For decision makers it is really great. We can really predict what is going to happen and you can make accurate decision. But, now imagine if there is no uncertainty, there are no tradeoffs to worry about, that means you are going to become redundant. Life may get boring. Imagine all your life paths are already predetermined. You may not even like it. So, we do want uncertainty in our life, and as an extension in business also. Because, uncertainty gives us opportunity to play and make some interesting choices, but the best way to do it is to come to a sort of agreement that says, uncertainty is useful. Have some meaningful discussions around uncertainty and then see how we can come up with good ways, in which we can reduce the domain of unpredictability. So, when we reduce the domain of unpredictability into the direction we want, some favourable outcomes occur. So, how to do that forms the crux of decision making in uncertainty.
"We need fast processing power as well as LLM methods and AI methods, to help you take decisions faster. So, don’t think that AI is there to replace you, because you will always take the final decisions"
-Prof. Jayendran Venkateswaran
Mohammed Sadiq: What do you think from the student point of view, if they need to relate and what would be the activities where this kind of decision making would come into play?
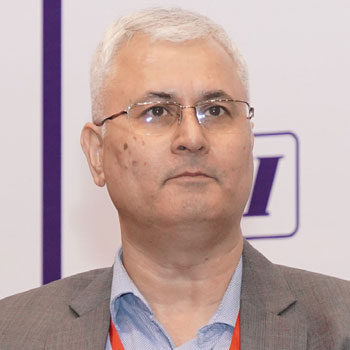
J Venkateswaran: Almost all the decisions that we end up taking, involves uncertainty. In many of the decision making courses that we study, the most common example is that we know the forecast. We know the manufacturer capacity, supplier capacity, everything is assumed to be known, and delivery times are known. But most of the time, this is actually not known. So, how actually can we think about all these problems. Broadly, we can think that there are some known unknowns and unknown knowns. I am not going to say about the other two extremes. What do I mean by these known unknowns? For example, when I say that the demand was uncertain. But, still people are making decisions on that—other interesting part which is very useful is like this unknown knowns. They are useful but we don’t know how to use them— that can be overcome by increasing visibility.
For example, this case came up when we worked with Indian Railways for spare parts management. There are millions of spare parts. But when you actually query them, you will find that same part may exist but is recorded very differently within the database. So you really don’t know whether you have those spare parts or not. These are unknowns but are knowns. You should know how many spare parts you have. But, sometimes it is very difficult to figure out where those spare parts are, so you end up ordering some more spare parts. How to increase this visibility? How to probe this further? We rely on technology to do that. For example, the most popular tool right now is GenAI or Large Language Models (LLMs). Can they look at the descriptions of some of these spare parts, so as to figure out whether they are the same spare parts or not? So that would be a kind of use case.
When we are looking at the transportation laytimes, say going from Mumbai to Goa, trucks are going to quote slightly different dates and exact arrival time becomes tricky depending on traffic conditions, so on and so forth. So the first thing we try to do is to combat it, to improve the visibility of actually where the truck is, how much goods it is carrying, which route it is taking, currently where it is? With higher visibility, we are able to reduce this domain of unpredictability and then use it to make better decisions.
Shammi Dua: From your experience and your interaction with the industry, which are the industry sectors or specific companies from which you would want to take examples and tell us how they are evolving in the use case for the use of decision-making methodologies, contemporary methodologies and tools? How do they stand?
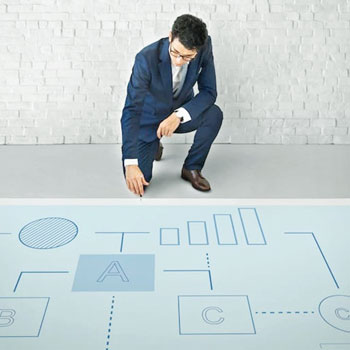
J Venkateswaran: Tata Steel has been one of the leading companies and have been in the forefront of technological development since a long time. Franz Edelman Award set up by the INFORMS Society in the US, is given to companies which are using latest analytics to improve their bottom line. The company must have a proven success model to become eligible for participating in this worldwide competition.
In 2024, Tata Steel and IIT Bombay worked on a project, where the former led it and we were only consulting. They implement steel annealing process where temperature control is very important to get the exact microstructures for producing steel of assured quality. Steel market is highly competitive. It was decided to create a model predictive control scheme, where the temperature is controlled using Math models. Earlier, it was run on every shift, and temperatures reduced every hour. Now it runs every minute. Every minute, the model is run real time, parameters are obtained and the feedback is provided. Based on temperature’s control, we are able to assure correct quality of steel is produced. This has resulted in 8 per cent reduction in CO2 emissions per ton of steel, which is really a large amount. As well as increase in 20 per cent in customer’s retention and sales aspects also. So, this is one example where they have used analytics to overcome uncertainty in the product quality.
Another example is from Airlines sector. American Airlines have developed Hub Efficiency Analytics Tool (HEAT). There are lots of flight disruptions due to weather. Traditionally, the companies used to focus only on the data they have, but now thanks to IoT and availability of lot of data, you can also get access to weather patterns upfront. So, they incorporated the inclement weather details for their hub operations, to proactively take decision on how should they schedule the flight, so that the passengers' waiting time is minimised before the inclement weather hits. After the inclement weather hits, you can’t do much, because the plane is grounded and it can’t take off. If you know it is going to hit, then can we have the planes moving around, so that they go to different places and passengers are moving. This way, you reduce the passengers’ waiting time. Once plane stops, all passengers are stranded, irate passengers are going to cost some money, pilots are stuck, everybody is stuck. So, they created this analytics tool to overcome this uncertainty, by using effective data which is beyond their boundaries. It’s not just the data about the pilots but going beyond. Can we leverage other data? For example, inclement weather data, what are operations elsewhere, what is the origin and destination of flights moving around, what is current customer movement from place A to B, and so on. Are we able to overcome this uncertainty of what’s going to happen if the weather hits?
Mohammed Sadiq: There are different ways and methods that can be utilised to leverage and get better outcomes. Could you explain three key methods or ways that we can look at, to improve and leverage for the outcomes to be better, for better decision making, and sunset (discontinuation of a product or service)?
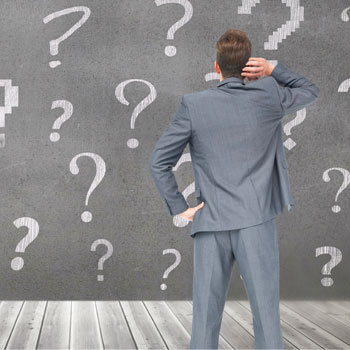
J Venkateswaran: First, we want to improve the visibility of information that we have, and that leads naturally to digitalisation, IoT, sensor networks, which give us tools and information, not only about our own operations but other data.
The second tool is the data analytics tools, which involves various types of models, including AI models and reinforcement learning models, we can use to improve our own. They are all decision support systems in my opinion. When these tools give the data, you have to interpret it and take decisions. Final decisions will rest with you, who are leading industrial experts and future managers.
"Don’t blindly trust the data or trust the outcome of the model. Please trust the data, or if you don’t trust then build some steps, so that you can build your own trust on the data that you have collected"
Mohammed Sadiq: If there is a connectivity that we can make from the legacy tools and methods like for example pareto analysis, fishbone method. Is there a possible connect we can establish between legacy and modern technology ways?
J Venkateswaran: I am an industrial engineer, so when I started my career, we started with pareto analysis, fishbone diagram, cause-effect analysis and so on. This understanding of cause and effect is very important even now. But the evolution of AI and those approaches if you see, they have become model free methods, where there is over reliance on only the data. But, we also have very good use cases which show that if we incorporate the domain knowledge within our AI models, their performance becomes much better. So, there is a natural reason for us to use some of these analysis to become part of this AI model that we are building, so that there is not only reliance on data but also some domain knowledge—all the traditional methods are also part of our analysis.
Shammi Dua: I can churn data with fast processors now, and do data analytics better and faster due to lowering data storage costs and improved internet speeds. What according to your observations, experience of interacting with different companies, could be an enabler or a showstopper from a faster adoption of these contemporary and upcoming tools?
J Venkateswaran: I have one pet view on this aspect that, now even some of these traditional legacy approaches are being peddled as an AI approach. Everything is packaged as AI, like AI insight that can potentially backfire. Because, suppose if that is already sold as AI and is found not to work so well, then companies will say we have already tried AI and it is not working. They will be dismissive and we will have to invent a new phrase, right now which is digitalisation and digital twinning. So, that is a kind of showstopper, because it is over reliance on the tool that is supposed to give the exact answer, which is not possible even if you think of any forecasting models or any approach. For example, ground team and sales team are expected to collect data and they also want some say in the decision making. So over reliance and saying that only this data will be used and everything is false, can be a kind of a showstopper.
But having said that, there are now very interesting education programmes where we are actually addressing this very nicely and saying that look we also need to know how to use the model, how to use the data, how to interpret the data, how to think in terms of recognising the uncertainty. So, uncertainty doesn’t mean that we only think of demand and randomness. Uncertainty can also come from delays and a long time.
For example, students here wouldn’t have planned for retirement, they are thinking about the placements. This is their maximum visibility. So the longer they go, the more uncertain it is going to be. There are lot of delays. You take some action and after some time only output comes out. So, that is also some form of uncertainty. We are trying to address all these things as this cannot be always anticipated.
So, getting involved in the modelling or the thought process, can increase productivity humongously. In example of railway spare parts, even for a pareto analysis or manual analysis, it will take months to prepare inventory statements and decide the quantities to be ordered. Some degree of automation can give the exact amount, but we want to spread it across the country. Imagine an organisation like Indian Railways which is trying to do for Pan-India or armed services—where there are millions of spare parts that has to be rotated between different times—we need fast processing power as well as LLM methods and AI methods to help take decisions faster. So, I want to tell the students here that don’t think AI is there to replace you, because you will always take the final decisions.
What we have to learn is how to use the extra information. At least people in 1990s knew, if there is a weather impact, it will impact flights, trains and so on. But, now we want to overcome that by getting accurate predictions. We want to look at the weather patterns. We can use weather predictions as they are becoming better. You have to bring in this ability—what other data can I use as part of Petrosian model, which can help me make better decisions. And, for analysing that, we have now supporting tools in the form of AI and others, to help us manage this uncertainty.
Shammi Dua: As a user of data, a lot of onus lies on me. We have heard this phrase “If you massage or punish the data long enough, it will start talking what you wanted to talk” very often. How do we control this bias where the user does not end up getting an output which he or she wanted?
J Venkateswaran: It is right that if you torture data long enough then it will confess to whatever you wanted to say. It’s a newer version of the old saying "Lies, damned lies and statistics". Bias happens on both sides, and one is a machine. There is always a danger of overfitting with the model, but at the same time we want to use lots of data. So, with actual appropriate methods— if you are able to justify why these have some sort of mental map or why these causal inputs should affect your outputs—you will be able to imagine mental model that can definitely go a long way in helping validate this. There is no automated way to say that you have overfit model or underfit model. Only thing I can say is, don’t blindly trust the data or the outcome of the model. Please trust the data, or if you don’t trust then build some steps, so that you can build your own trust on the data that you have collected.
"If you have to handle uncertain ty, then you must be able to quantify it. We do take decision making under uncertainty regularly"
Mohammed Sadiq: If there are some five skills that the young professionals and students need to upskill themselves with, to become much better decision makers in times of uncertainties then what would those skills be?
J Venkateswaran: First skill is systems thinking skill. Before you embrace uncertainty, we must become comfortable, able to think and connect the different paths of the system, and able to see the causal map of how the system evolves. Like for example, we are talking about supply chain—there are many players in the supply chain. Mentally picture—if the sales team is going to increase their advertising efforts, then what kind of impact are you expecting at the supplier side, maybe two months hence? So, one is ability to first do that. There are very good basic models that are available. There are many models and mathematical concepts which you can use to exercise and build your own intuition, which I would say, you start from there. You will get a feel of what is happening and what happens, due to the impact of delays, long laytime, and feedbacks can be thought of. If you have to handle uncertainty then you must be able to quantify it.
We do take decision making under uncertainty regularly. For example, you need to catch a train from Pune station that is supposed to leave at 4 pm. Even if Google says you can reach Pune station in 50 minutes, you won’t leave at 3 pm, but you will still leave at 2.30 pm or 2 pm. This means, you are already hedging against worst case scenario. Reasonable, but you will not go at 8 am in the morning. Other thing is, we are comfortable about the mean performance—on an average it should work. But, when you do on an average, then 50 per cent of the times you may be wrong. So, some skills of understanding this breadth variance with some knowledge of statistics and data analytics, will really go a very long way. There are many analytics tools, but I would say just focus on variance. One favourite mathematical concept is co-efficient of variance. If you get a feel of how much is variability compared to mean performance, then much of the uncertainty can be nicely managed. See how we can reduce it with more and more information.
"If you have to handle uncertainty, then you must be able to quantify it. We do take decision making under uncertainty regularly"
Mohammed Sadiq: So, the key is to take theoretical and book study into practical life every day in and out, so that you bring that into mode where uncertainty falls and you take right decisions on the go.
J Venkateswaran: True. The other one is classical inventory approach, which is called as news vendor model. Many of you might have already heard of it. You are wondering already the book is there, some math model is there, we will use it. But, the model actually is not trying to force you to do that. It is like saying, even though there is uncertainty, demand is not known, but some price and cost is there, and just going with the mean demand, may not work. You may either need to go less or more, depending on the price of the products—that is one. And number two, nobody is saying that you have to use only one distribution of demand, no need.
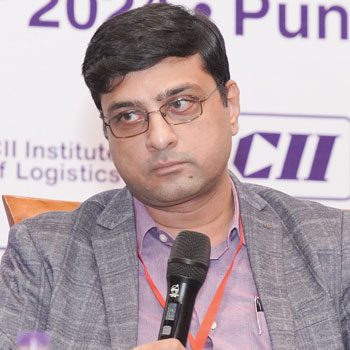
Now with AI analytics tools, you can update your demand pattern every day. For every product, you can make demand patterns. For every season, you can make demand patterns. You can make every Monday a demand pattern. Before Dussera and before Diwali, this is a demand pattern. So, instead of having one demand pattern, which is what the textbook will tell you that is not learning. Learning is, if I know something about demand pattern, then I can plot a histogram. Histogram gives you what kind of proportion it is going to occur. But, since I have a large amount of data with large number of products, I can use tools of AI and data analytics, to find that data is forming one pattern or is forming another pattern.
Sometimes you don’t need expert knowledge to get data. Experts will say, on Monday morning banks are busier. But, you don’t need expert to tell it. Now you can have many different distributions that already tell you how to handle uncertainty. If you are able to get a better prediction of the distribution, I can use models which are reasonably well. On a long term you will make more efforts, but these small improvements can squeeze something more, make the system little more efficient. For example, Indigo Airlines - there is a lot of uncertainty in the turnaround of an aircraft. So, what did they do? They have two exit gates and a nice ramp. Some people walk slowly on steps, and people get stuck. So, a ramp is there to ensure that the exit time of everybody is fairly constant. Wheelchairs are already there. There are multiple buses to evacuate people immediately. Next round of passengers are already waiting. So, the turnaround time of the aircraft can be reduced. But first for us, let us understand that there is some variability, there is some uncertainty in the turnaround time. Can I reduce it? As soon as I reduce it, I can have one extra flight that day, which can be a huge game changer.
This does not mean that a student must use textbook examples and anecdotes, only when a particular scenario occurs. They are illustrations and you need to find the correct concept for using it. AI is not going to come up with new concepts. After using AI to crunch it, you can use it to put in the news vendor model, which gives pretty good results. You can see the difference in your bottom line. Even now, EOQs and news vendor model continues to be relevant. Except that, now you get a wonderful opportunity to update the data very frequently that makes a huge difference in managing uncertainty.